Bayesian adjustment for misclassification in cancer registry data
Introduction
Cancer is a burgeoning health problem worldwide, and poses an increasing burden for lots of countries in the world. Mortality and incidence are the familiar projections in the assessment of the burden of cancers. With regards to cancer data registry in countries, data are important to monitor the effects of screening programs, earlier diagnosis and other prognostic factors (1). Data on cancer mortality can be used to guide policy makers in order to setup cancer prevention programs. But this aim needs completed reliable death registry systems which report death statistics annually and accurately. On the other hand, the analysis of death statistics subject to misclassification, is a major problem in epidemiological analysis, often leading to biased estimates, and can therefore cause one to underestimate health risks (2). The World Health Organization (WHO) has encouraged member states to introduce systems of death registration involving medical certification of the cause of death. But for developing countries, the registration system almost could not cover the total population or subjects to misclassification.
In statistical literature, two approaches are recommended, when misclassification occurs. The first: using a small validation sample (3) and the second: Bayesian analysis in which subjective prior information on at least some subset of the parameters is used to re-estimate misclassified statistic (4,5).
Similar to other developing countries, Iranian mortality information is still incomplete (6). According to the Iranian death registry, between 15% to 20% of death statistics are recorded in misclassified categories such as septicemia, Senility without mention of psychosis Symptoms, and other ill-defined conditions, etc. (7). This problem happened for mortality data of Gastrointestinal (GI) cancers too. GI cancers are the most frequently occurring cancer among Iranian males and second only to breast cancer among females (8-10). In fact, GI cancers account for nearly half (44.4%) of all cancer related deaths in Iran (11). Recently we developed a Bayesian model to correct the misclassification in GI cancer mortality data and applied this model on mortality of colorectal cancer (CRC) (12), liver cancer (13), gastric cancer (14), esophageal cancer (15) and oral cavity cancer (16). The aim of this study was to explain this Bayesian model and provide quantitative estimations of the burden of death in term of years of life lost’ (YLL) due to CRC in Iran after re-estimation of death with this Bayesian approach.
Materials and methods
Data sources
The National Organization for Civil Registration (NOCR) and the Ministry of Health and Medical Education (MOH&ME) have established death registration systems in Iran (6). National death Statistics Reported by the MOH&ME from 1995 to 2000 (registered death statistics for Iranian population at the Information Technology and Statistic Management Center, MOH&ME) and from 2001 to 2004 (published by MOH&ME) (7,11,17) stratified by age group, sex, and cause of death [coded according to the 9th revision of the International Classification of Diseases (ICD-9)] are included in this analysis. CRC mortality (ICD-9; 153-154) expressed as the mortality rate for each 100,000 people. The incidence rate was obtained from the reported results of cancer registry of MOH&ME. The populations of Iran in 1995-2004 were estimated by age group and sex using the census from 1996 conducted by Statistics Centre of Iran and its estimation according to population growth rate for years before and after national census. In order to facilitate the quantification of diseases in the case of mortality, YLL was employed (1,18,19). YLL is the number of years which would be saved in the absence of the disease. Because mortality does not directly reflect the issue of premature death, YLL provides a more accurate depiction of premature death by weighting deaths occurring at younger ages more heavily than those occurring in older populations.
Statistical implementation
The Bayesian approach considered here was derived from models proposed by Stamey et al. to correct and account for misclassification in a Poisson regression (2). Stamey’s technique extended the model to overcome the problem of misclassification in cancer data (4,5) and Pourhoseingholi et al. developed this technique to estimate mortality rate of GI cancers (12-16). Suppose we have two sample groups for death classification; and
where r is the covariate pattern, y1 is the exact cause of death and y2 is the misclassified group in which the cause of death in the first group was incorrectly labeled, and y1~ Poisson (Piμi1) and y2~ Poisson (Piμi2) in which μi is the observed rate of death mortality for the covariate pattern. Let θ be the probability that an observation from group 1 is incorrectly labeled as belonging to group 2. If the actual rate of death for each group (unknown) is supposed to be as λi, the relation between actual rate and observed rate can be written in following form; μi1 = λi1(1–θ) and μi2 = λi2 + λi1θ.
The joint distribution of the observable mortality data in this case of misclassification is proportional to:
To perform Bayesian inference, we assume that informative beta prior distribution for the misclassified parameter, i.e., θ ~ beta(a,b). Because θ is an unknown parameter, we employed a latent variable approach according to Paulino et al. (20,21), Liu et al. (22) and Stamey et al. (2) to simplify the full conditional models and estimate the posterior distribution using a Gibbs sampling algorithm. In this case, we define Ui|β1,β2,θ,y1,y2~ Binomial (yi2,Pi) to be the number of counts from the first group incorrectly labeled as being in the misclassified group. So; and finally the posterior appears in the following form:
The misclassification probability estimate which is proposed in prior distribution was based on Iranian death registrations which introduced between 15% to 20% of misclassified records into total deaths. We assumed a 20% misclassification with a beta prior to re-estimate the death statistic related to CRC from misclassified groups. All analysis were performed by a Macro and developed in S-Plus.
Results
Mortality data consisting of all deaths due to CRC from 1995 to 2004, (up to 7,548 records) were considered in this study. YLL due to CRC, classified by sex and age, generated from original database (Frequentist estimation) and their Bayesian corresponding projections (Bayesian estimation) appeared in Table 1 and Figure 1. According to the Bayesian estimation there were between 30% to 40% percent underestimation for YLL due to CRC. The burden of CRC increased constantly from 1995 to 2003 but decreased again in 2004. Also YLL due to CRC was higher for older age (Table 1). Figure 2 showed YLL for CRC and its Bayesian estimation according to gender, indicating that YLL for male was high comparing to female considerably.
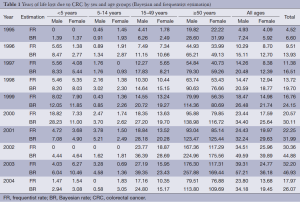
Full table
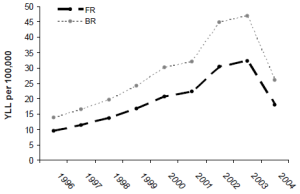
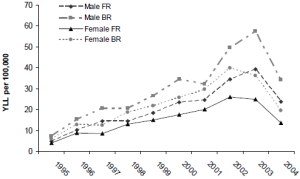
Discussion
Our results indicated that between 30-40% of YLL due to CRC remains underreported and suggest a substantial undercount of CRC burden in the Iranian population. The instrument YLL has been widely used for calculating the burden of diseases due to premature death and in health planning (1,18,19). Response misclassification of counted data for death statistics is still a problem in developing counties. Analysis of YLL depends on mortality data but misclassification in death statistics leads to biases and underestimates.
Mortality is an important input for calculating the YLL, so it is obvious that, in the case of misclassification, in which the death statistics subject to underestimation, the YLL would be underestimated too. Similar to other developing countries, Iranian mortality information is still incomplete (6) and between 15% to 20% of death statistics are recorded in misclassified categories (7). In the new Iranian Death Registration System, data on causes of death are collected from various sources and have been assessed to be about 80% complete (6). In spite of this new registry system, there is still up to 20% undefined death records that categorized as misclassification.
Recently Bayesian approach received much attention in the case of misclassification. McInturff et al. used a Bayesian approach to estimate the parameters of a binomial regression with misclassification (23). Whittemore and Gong used this approach to estimate cervical cancer mortality rates (4) and Sposto et al. developed this likelihood to assess the effect of diagnostic misclassification on non-cancer and cancer mortality dose-response (5). Stamey et al. used Bayesian approach in data consisting of the number of deaths due to cancer and non-cancer among residents of Hiroshima and Nagasaki, Japan (2) and we studied this technique to estimate mortality rate in GI cancers (12-16) according to Iranian death statistics.
Our study indicated that although the YLL due to CRC seems to be low; up to 40% of this measurement was underestimated. In addition the increase of YLL during these recent years (ignoring a dropped in 2004) and predicting to experience a higher incidence in future indicated this fact that the population may be experiencing an acceleration of the disease burden (24,25). In Iran comparing to west, most of CRC patients diagnosed in younger age (26) tumors appearing at earlier ages and resulting in death are clearly associated with greater lost years of life (27,28).
In our study men had greater YLL than women. This finding is in agreement with other studies that suggested greater burden of CRC for men (28).
Conclusions
Our findings suggested a substantial underestimated YLL due to CRC in Iranian population. So healthcare policy makers who determine research and treatment priorities on death rates as an indicator of public health priorities should notice to this underreported data, specifically in the countries with incomplete registry data.
Acknowledgements
This study was sponsored by a grant from the Gastrointestinal and Liver Disease Research Center, Shahid Beheshti University of Medical Sciences, Tehran, Iran.
Disclosure: The author declares no conflict of interest.
References
- Burnet NG, Jefferies SJ, Benson RJ, et al. Years of life lost (YLL) from cancer is an important measure of population burden--and should be considered when allocating research funds. Br J Cancer 2005;92:241-5. [PubMed]
- Stamey JD, Young DM, Seaman JW Jr. A Bayesian approach to adjust for diagnostic misclassification between two mortality causes in Poisson regression. Stat Med 2008;27:2440-52. [PubMed]
- Lyles RH. A note on estimating crude odds ratios in case-control studies with differentially misclassified exposure. Biometrics 2002;58:1034-6; discussion 1036-7. [PubMed]
- Whittemore AS, Gong G. Poisson regression with misclassified counts: application to cervical cancer. J R Stat Soc Ser C Appl Stat 1991;40:81-93. [PubMed]
- Sposto R, Preston DL, Shimizu Y, et al. The effect of diagnostic misclassification on non-cancer and cancer mortality dose response in A-bomb survivors. Biometrics 1992;48:605-17. [PubMed]
- Khosravi A, Taylor R, Naghavi M, et al. Mortality in the Islamic Republic of Iran, 1964-2004. Bull World Health Organ 2007;85:607-14. [PubMed]
- Naghavi M. Death report from 29 provinces in Iran. 1st edition. Tehran: Ministry of Health and Medical Education, 2007.
- Mosavi-Jarrahi A, Mohagheghi MA. Epidemiology of esophageal cancer in the high-risk population of iran. Asian Pac J Cancer Prev 2006;7:375-80. [PubMed]
- Pourhoseingholi MA, Vahedi M, Moghimi-Dehkordi B, et al. Burden of hospitalization for gastrointestinal tract cancer patients - Results from a cross-sectional study in Tehran. Asian Pac J Cancer Prev 2009;10:107-10. [PubMed]
- Pourhoseingholi MA, Vahedi M, Pourhoseingholi A, et al. Comparing linear regression and quantile regression to analyze the associated factors of length of hospitalization in patients with gastrointestinal tract cancers. JPH 2009;6.
- Naghavi N. Death report from 23 provinces in Iran. 1st edition. Tehran: Ministry of Health, 2004.
- Pourhoseingholi MA, Faghihzadeh S, Hajizadeh E, et al. Bayesian estimation of colorectal cancer mortality in the presence of misclassification in Iran. Asian Pac J Cancer Prev 2009;10:691-4. [PubMed]
- Pourhoseingholi MA, Fazeli Z, Zali MR, et al. Burden of hepatocellular carcinoma in Iran; Bayesian projection and trend analysis. Asian Pac J Cancer Prev 2010;11:859-62. [PubMed]
- Pourhoseingholi MA, Faghihzadeh S, Hajizadeh E, et al. Bayesian Analysis of Gastric Cancer mortality in Iranian Population. Gastroenterol Hepatol Bed Bench 2010;3:15-18.
- Pourhoseingholi MA, Abadi A, Faghihzadeh S, et al. Bayesian analysis of esophageal cancer mortality in the presence of misclassification. Ital J Public Health 2010;8:342-47.
- Pourhoseingholi MA, Vahedi M, Baghestani AR, et al. Bayesian correction for mortality trend of oral cavity cancer. Gastroenterol Hepatol Bed Bench 2012;5:S8-12. [PubMed]
- Naghavi M. Death report from 18 provinces in Iran. 1st edition. Tehran, Iran: Ministry of Health and Medical Education, 2003.
- Gardner JW, Sanborn JS. Years of potential life lost (YPLL)--what does it measure? Epidemiology 1990;1:322-9. [PubMed]
- Mariotti S, D’Errigo P, Mastroeni S, et al. Years of life lost due to premature mortality in Italy. Eur J Epidemiol 2003;18:513-21. [PubMed]
- Paulino CD, Soares P, Neuhaus J. Binomial regression with misclassification. Biometrics 2003;59:670-5. [PubMed]
- Paulino CD, Silva G, Achcar JA. Bayesian analysis of correlated misclassified binary data. CSDA 2005;49:1120-31.
- Liu Y, Johnson WO, Gold EB, et al. Bayesian analysis of risk factors for anovulation. Stat Med 2004;23:1901-19. [PubMed]
- McInturff P, Johnson WO, Cowling D, et al. Modelling risk when binary outcomes are subject to error. Stat Med 2004;23:1095-109. [PubMed]
- Ansari R, Mahdavinia M, Sadjadi A, et al. Incidence and age distribution of colorectal cancer in Iran: results of a population-based cancer registry. Cancer Lett 2006;240:143-7. [PubMed]
- Pourhoseingholi MA, Zali MR. Colorectal cancer screening: Time for action in Iran. World J Gastrointest Oncol 2012;4:82-3. [PubMed]
- Moghimi-Dehkordi B, Safaee A, Zali MR. Prognostic factors in 1,138 Iranian colorectal cancer patients. Int J Colorectal Dis 2008;23:683-8. [PubMed]
- National Cancer Institute. Surveillance, Epidemiology and End Results. SEER Cancer Statistics Reviews (1973-1997) [electronic citation] 2008. Available online: http://www.seer.cancer.gov
- Perez-Palma J, Marchena-Gomez J, Dorta-Espineira M, et al. Predictive factors of years of potential life lost by colorectal cancer. Eur J Gastroenterol Hepatol 2008;20:766-72. [PubMed]